データを活用することにより我々がこの世界を、この宇宙をもっとよく理解できるようにしたいと考えています。そのためにはデータから有益で正確な情報を抽出する必要があります。既存の科学技術はそのための十分な術をすでに与えてくれているでしょうか?私の考えではそれはまだ十分ではなく研究の余地が十分にあります。私はこの目的のための研究を行なっています。
因果を見つける
取り組んでいる研究の大きなテーマとして観察データからの因果の推論というものがあります。因果についての統計学的研究は、学術的に非常に難しいタスクであると認識されており、現実にある多種多様なデータへの適用とその活用にもまだかなりの隔たりがあると考えられ、精度そして汎用性ともに高いメソッドやアルゴリズムの研究を実施してきました。
その研究成果を因果分析のソフトウェアとしてツール化し、その実データへの適用を2014年から開始しています。その後CALCプロジェクトとして本社部門とも協力しソニーグループ企業群に展開しています。現在では日米欧におけるエレキ、エンタメ、金融、その他サービスを含む広範なソニーのデータ分析で活用されるようになっており、多くの協力メンバーや賛同者とともにソニーのデータ分析業務の変革に取り組んでいます。この成果を受け、外部へのソフトウェアライセンス販売や受託分析も外部の企業と共同で行ない製造業やサービス業などの様々な分野で利用されています。
この研究の根底にも、データから世界を、宇宙をより理解できるようにしたい、そのために因果関係をデータからもっと識別できる方法の発展が必要だという考えがあります。また、この領域の基礎科学の観点からの興味深い点としてそれが統計学に閉じていないという面があります。科学哲学や物理学とも関係していると考えられ、その方面での考察も進めています。推論メソッド・アルゴリズムや因果情報の活用に関する研究は、未開拓の大きな課題や、実際の活用の過程で新しく見つかる重要な課題もあり、プロジェクト研究員も加わって進めており、データからの推定・推論の限界に挑んでいます。また、他の学問分野への応用も考え、いくつかの大学との共同研究も進めています。
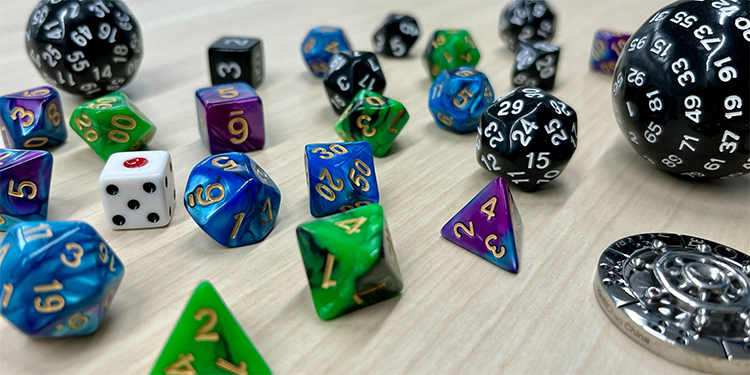
推定の基礎原理を探る
取り組んでいる他の研究の1つには、非常に基本的なのですが、データからの確率や統計モデルの推定に関する考え方について、というテーマがあります。通常確率の推定は、日常の言葉で言えば割合、やや専門的な言葉で言うところの相対頻度、として計算されるということは皆さんの日常生活にも十分馴染んでいるだろうと思います。この相対頻度からなる確率の推定計算は統計学的には尤度という量が最大となるような推定量にするという原理をバックボーンとしています。頻度主義とも言われ統計学では最も基盤となる考え方です。ところが任意のサンプル数で成り立つものではなく、特に少数のサンプルでは信頼性が大きく落ちてしまいます。つまり尤度最大の原理はある種の近似であると考えることができます。そこで筆者は、より汎用的な原理があってもいいのではないかと考えて研究を始め、そこである原理が思い浮かびました。それは物理学における熱力学に基づく考え方であり、自由エネルギー最小原理と呼ぶべきものです。この考え方は2007年に萌芽的研究として発表したあと、精緻化を試みるほか因果の推論メソッドにも活用しています。この原理の統計科学への適用は確率の推定からモデルの選択まで統一的に議論できる可能性がある点でも優れていると考えています。またこの考え方が適用できるのであれば、有限のデータから情報を抽出する世界において、物理学的世界との類似性を議論することにも繋がるため、科学哲学の世界としての深みを持っていると考えています。また、この考え方は実はほぼ同時期に脳科学においても類似し(てはいるが異なっ)た原理が提唱されているほか、AIの大規模言語モデルにおいても私がこの原理に関係して注目している考え方がテクニックとして活用されていることなどもあり、さらに追求する価値が高まっていると考えています。
We aim to better understand our world, and even the universe, by utilizing data. To achieve this, it's crucial to extract useful and accurate information from data. The question then arises: does current science and technology provide us with sufficient means for this purpose? In my opinion, it is not yet sufficient, and there is still ample room for research. I conduct research for this purpose.
Finding Causation
A major theme of my research is the inference of causality from observational data. Statistical studies on causality are acknowledged as academically challenging tasks. It is believed that there is still a considerable gap in applying and utilizing these studies to the wide variety of data available in reality. Consequently, I have been conducting research on methods and algorithms that are both accurate and highly versatile.
The outcome of the research has been developed into a software tool for causal analysis, which began being applied to real data in 2014. This initiative has since expanded within the Sony Group as the CALC project, in cooperation with our headquarters' division. It is now utilized across a wide range of Sony's data analyses, including in the electronics, entertainment, finance, and other service sectors in Japan, the United States, and Europe. Together with many cooperating members and supporters, we are working to transform Sony's data analysis operations. Based on these results, the software license sales to external parties and commissioned analysis are also conducted in collaboration with external companies and the system is utilized in various fields in the manufacturing and service industries.
At the heart of this research lies the aspiration to deepen our understanding of the world and the universe by enhancing our ability to discern causal relationships from data. This necessity for the development of methods to more accurately identify causation from data is a driving force behind our work. An interesting aspect of this field from the perspective of basic science is that it extends beyond the confines of statistics alone. It is believed to intersect with the philosophy of science and physics, and we are pursuing considerations in these areas as well. Our research on inference methods, algorithms, and the utilization of causal information addresses both vast unexplored challenges and significant issues that emerge during practical application. The project includes researchers working together to confront the limits of estimation and inference from data. We are also exploring applications in other academic disciplines and advancing collaborative research with several universities.
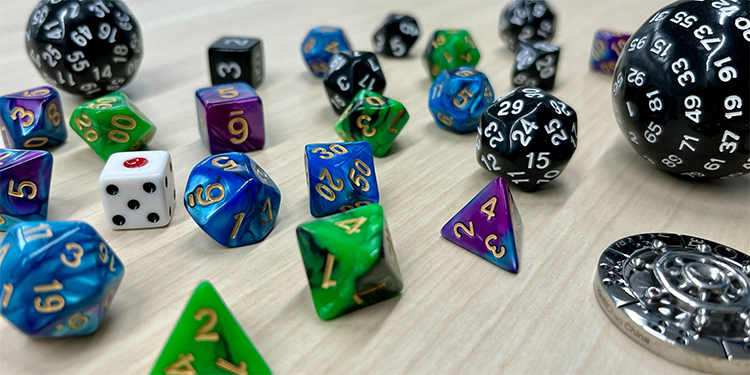
Exploring the Fundamental Principles of Estimation
One of the other research topics I am working on, though very fundamental, concerns the concepts related to the estimation of probabilities and statistical models from data. It is commonly understood that probability estimation, in everyday terms referred to as a ratio or, in slightly more specialized language, as a relative frequency, is well integrated into our daily lives. The principle of maximizing the likelihood, which means making the estimation such that the quantity known as the likelihood in statistics is maximized, underpins the calculation of probability estimates based on this relative frequency. This principle, known as frequentism, forms the foundation of statistical thinking. However, it does not hold for any sample size and is particularly unreliable with a small number of samples. In other words, the principle of maximum likelihood can be considered a type of approximation.
With this in mind, I began researching the possibility of a more universal principle and was inspired by a concept based on thermodynamics in physics, which should be referred to as the principle of minimum free energy. This idea, initially proposed as an embryonic study in 2007, has since been refined and applied to methods for inferring causality. The application of this principle to statistical science is promising because it allows for a unified discussion from the estimation of probabilities to model selection. Moreover, if this concept is applicable, it could bridge the physical world with the extraction of information from finite data, suggesting a profound connection to the philosophy of science. Interestingly, a similar (yet distinct) principle was proposed in neuroscience around the same time, and related ideas have been utilized in techniques for large-scale language models in AI, underscoring the increasing value of further exploration in this area.