Takashi Isozaki
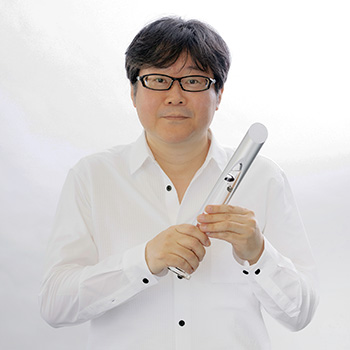
We are now using more data than ever before, and this usage is sure to go on increasing in the future. Many examples of mass-data can be listed, such as data related to geoenvironmental assessments, gene expressions, and buying history. It is easy to imagine that success in utilizing such data would have a great influence on the future of humanity. However, humankind does not currently have the ability to fully extract information from data, which I believe is a major problem. I have addressed this problem by treating data that have many variables dependent on each other using probabilistic graphical models and methodologies of physics.
Selected Publications
Isozaki, T. and Kuroki, M. (2017), Learning Causal Graphs with Latent Confounders in Weak Faithfulness Violations, New Generation Computing, Vol. 35, Issue 1, pp. 29-45.
Funabashi, M., Hanappe, P., Isozaki, T., Maes, A.M., Sasaki, T., Steels, L. and Yoshida, K. (2015), Foundation of CS-DC e-laboratory: Open Systems Exploration for Ecosystems Leveraging, Proceedings of CS-DC 2015, Springer.
Isozaki, T. (2014), A Robust Causal Discovery Algorithm against Faithfulness Violation, Trans. of the Jpn. Soc. for AI, Vol. 29, No.1, pp. 137-147.
Worldviews
A big Data Analysis Tool
Keywords
News & Articles
Practically effective adjustment variable selection in causal inference
Challenging the Limits of Information Extraction from Data
Adjustment Variable Selection for Intervention Estimation Robust to Data Size