Project from Social Implementation
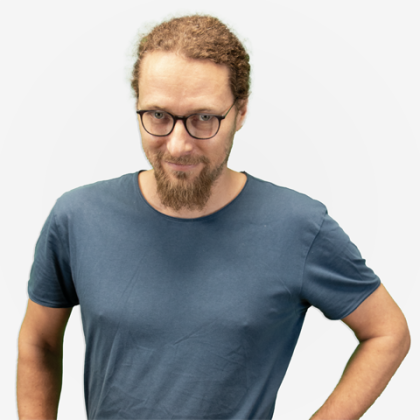
Music is an artistic discipline and as such allows for a wide variety of realizations. Still, in actual practice, it is by no means a case of "anything goes." Music is highly structured and obeys specific rules. In addition, it is still not fully understood why some musical structures are better perceived by an audience than others. All that makes it a fascinating topic for research, both for analysis and generation. Finding patterns in music (the "what") can be technically challenging, and the different types of patterns result in diverse specializations in the field of music information research. Such patterns provide evidence for an even more challenging and fascinating question: Why is music structured in such ways, and why does the same music appeal differently to different individuals? As music does not need logical thinking to be appreciated, it can be well-described by mere statistical methods. Machine learning is inherently statistics and therefore a well-suited paradigm to answering such questions.
Studying music analysis and generation with computational methods allows for approaching a holistic understanding of the matter. I am convinced that one can only really understand something if one can reproduce it. For me, this means focusing on music analysis, music making ("musicing") and music generation. The fortunate compatibility of music making and music generation leads directly to my main research direction of AI-assisted music making, and I strive to develop tools that help make the creation of music more intuitive, enjoyable, and direct.